The use of artificial intelligence (AI) has become increasingly common across many different industries seeking to make processes smarter and more efficient. The wastewater industry is no exception. Discussion about the roles AI can and will play in assessing and managing wastewater assets has been growing as the technology evolves.
One of the areas AI is expected to make a big impact is in the sewer inspection process, through the use of image recognition. In the world of AI, image recognition has the ability to “see” the images it collects. With machine learning techniques, computers can be trained to identify and categorize objects they “see” in the images and video frames. This means image recognition can aid wastewater operators in automatically locating and classifying defects in a pipe.
There are several ways AI can be used to assess inspection footage and scan for defects, including object detection and image segmentation.
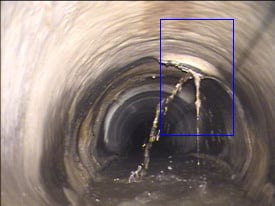
Object detection has two main goals: identify the objects in an image and locate them. Object detection places rectangular “bounding boxes” around the object or objects and a label of what the AI predicts it is based on input data.
Using this method, the operator receives a list of bounding boxes defined by their location—using coordinates with the top left corner of the image as the starting point—and a label indicating what’s been detected. Operators can refer to these bounding boxes to closer identify the defects or abnormalities picked up by AI.
However, this method has some clear limitations. Not all defects are rectangular in shape, so the bounding box is a best-fit method and does not give information about the shape or curvature of the object.
Image segmentation uses a pixel-by-pixel approach to assess an image and scan for defects. Surveying the picture by each individual pixel, image segmentation generates a much more detailed and precise model of the defect or object identified.
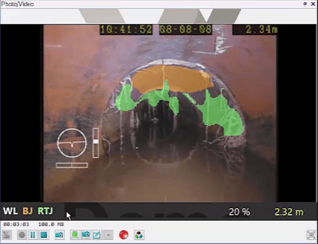
The output is a mask image the same size as the original, but the pixels with detected defects are highlighted in a different color than the rest of the image. This method provides data about the size and shape of each defect.
There are two techniques of image segmentation: semantic segmentation and instance segmentation. Semantic segmentation detects objects and groups them based on a defined category. For example, each crack identified in the pipe may be labeled in red. Instance segmentation goes more in-depth to classify the objects within the defined categories. For example, a longitudinal crack may be labeled in blue while a circumferential crack is labeled in red, etc.
Using AI, both object detection and image segmentation offer a means for identifying the presence of a defect in an image, which can aid the operator in faster, and potentially more accurate inspections. However, the information the operator is looking to gain from the inspection may determine which method is preferable. Object detection is helpful in determining whether an object is present or not. Image segmentation determines whether or not an object is present and also provides information about the shape, size and measurements of the defect.
WinCan’s new AI toolkit, Sewermatics, automatically detects defects in video inspections using image segmentation, assigns defect codes and auto-populates data like size, position and water level in 30 different languages. Sewermatics will be available across WinCan’s full lineup of inspection reporting and asset management solutions, including WinCan VX, WinCan Embedded and WinCan Web. Learn more AI's impact on collection system inspection and maintenance by downloading WinCan's free white paper: